Introduction
The field of protein folding, a fundamental aspect of understanding the structure and function of proteins, has long been a challenge in biomedical research. Proteins, the building blocks of life, play critical roles in nearly every biological process, from enzyme catalysis to immune response. Understanding how proteins fold into their native three-dimensional structures is essential for deciphering their functions and designing novel therapeutics. However, traditional methods for predicting protein structures have been labor-intensive and time-consuming. Enter artificial intelligence (AI), which is revolutionizing protein folding prediction and unlocking new frontiers in biomedical research. In this article, we’ll explore how AI is transforming protein folding prediction, its implications for drug discovery and personalized medicine, and the future of this exciting field.
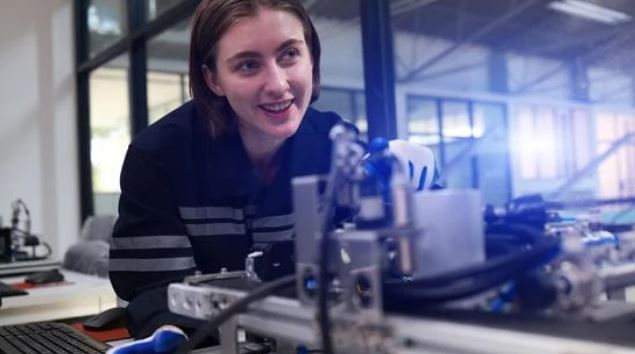
Understanding Protein Folding
Proteins are linear chains of amino acids that fold into specific three-dimensional shapes, dictated by their amino acid sequence. The process of protein folding is complex and occurs spontaneously as proteins navigate a vast conformational space to reach their native, functional structures. However, predicting how a protein will fold based solely on its amino acid sequence has proven to be a daunting task, requiring significant computational resources and experimental validation.
The Role of AI in Protein Folding Prediction
AI, particularly machine learning and deep learning algorithms, has emerged as a powerful tool for predicting protein structures with unprecedented accuracy and efficiency. These algorithms analyze vast amounts of protein sequence and structural data to learn patterns and relationships, enabling them to make accurate predictions about how a given protein will fold. By leveraging AI, researchers can accelerate the process of protein structure prediction and gain insights into protein folding mechanisms that were previously inaccessible.
Benefits of AI-Powered Protein Folding Prediction
- Speed and Efficiency: AI algorithms can rapidly analyze protein sequences and predict their structures in a fraction of the time required by traditional methods. This acceleration enables researchers to study a wider range of proteins and accelerate the pace of discovery.
- Accuracy and Precision: AI-driven protein folding prediction methods have demonstrated remarkable accuracy, rivaling or surpassing traditional experimental techniques. These predictions provide valuable insights into protein structure-function relationships and aid in drug discovery efforts.
- Cost-Effectiveness: By reducing the need for expensive and time-consuming experimental techniques, AI-powered protein folding prediction offers a cost-effective approach to studying protein structure and function. This cost savings enables researchers to allocate resources more efficiently and pursue innovative research avenues.
- Scalability and Accessibility: AI algorithms for protein folding prediction are scalable and accessible, making them suitable for researchers of all levels of expertise. These algorithms can be deployed on cloud-based platforms, allowing researchers to access powerful computational resources without the need for specialized hardware.
Applications of AI in Biomedical Research
- Drug Discovery and Development: AI-powered protein folding prediction is revolutionizing drug discovery by enabling researchers to identify novel drug targets and design more effective therapeutics. By understanding the structures of target proteins and their interactions with potential drug molecules, researchers can accelerate the development of new drugs for a wide range of diseases.
- Personalized Medicine: AI algorithms are facilitating the development of personalized medicine approaches by enabling researchers to predict how individual variations in protein sequences may impact protein folding and function. This information can guide treatment decisions and improve patient outcomes by tailoring therapies to the unique characteristics of each patient.
- Disease Diagnosis and Biomarker Discovery: AI-driven protein folding prediction methods are also being used to identify disease-associated protein structures and biomarkers. By analyzing protein sequence and structural data from patients with various diseases, researchers can identify patterns and signatures that may serve as diagnostic markers or therapeutic targets.
- Biotechnology and Bioengineering: In addition to biomedical research, AI-powered protein folding prediction has applications in biotechnology and bioengineering. By designing proteins with specific structures and functions, researchers can develop novel enzymes, biomaterials, and biotherapeutics with a wide range of industrial and medical applications.
Challenges and Future Directions
While AI has shown great promise in protein folding prediction, several challenges remain. One of the main challenges is the need for large and diverse datasets to train AI algorithms effectively. Additionally, AI algorithms may struggle with proteins that exhibit complex folding dynamics or require post-translational modifications. Addressing these challenges will require interdisciplinary collaboration between computer scientists, biophysicists, and bioinformaticians.
Looking ahead, the future of AI in protein folding prediction holds immense potential. Advances in deep learning algorithms, coupled with the availability of high-quality protein structure data, will continue to improve the accuracy and efficiency of protein folding prediction methods. Furthermore, integrating AI-driven protein folding prediction with experimental techniques, such as cryo-electron microscopy and X-ray crystallography, will enable researchers to validate and refine computational predictions, leading to a deeper understanding of protein structure and function.
Conclusion
AI-powered protein folding prediction is revolutionizing biomedical research by unlocking new frontiers in our understanding of protein structure and function. By leveraging AI algorithms, researchers can predict protein structures with unprecedented accuracy and efficiency, accelerating drug discovery, personalized medicine, and biomarker discovery efforts. While challenges remain, the future of AI in protein folding prediction looks promising, with potential applications across a wide range of fields, from biotechnology to healthcare. As AI continues to evolve, so too will our ability to unlock the secrets of protein folding and harness its power for the benefit of human health and well-being.